Emotion EEG Data-set
In this study, four basic emotional states are examined during the audio-video stimulus. The 20 healthy subjects of age group (22.5 ±2.5) have participated in the experiments, who were the undergraduates of PDPM, Indian Institute of Information Technology, Design and Manufacturing, Jabalpur. For evoking the subjects emotional states, the audio-video clips of Indian films are used as elicitors. The clips are selected based on the following criterions. (i) A clip should be relatively short to avoid the contaminated data recording of multiple emotions. (ii) The clip should be understood by subjects without any explanation. (iii) The clip should elicit a single targeted emotion. To further assess that the selected clips evoked the emotion properly or not, the questioner session on 60 volunteers is performed. The selected volunteers are not included in the final EEG recordings to assess the efficacy of selected elicitors (clips) for inducing the emotions. In the questioner session, 30 movie clips are shown to volunteers and they were asked to rate these from 1–10 ratings in four emotions categories. The three higher mean rating clips for each emotion are selected. The experiment is begun with providing the experiment manual to subjects, which explained the experimental procedure and rating scales for assessing the evoked emotional state. The induced emotional states are following the 2-D valence-arousal emotion model, including happy (high arousal and high valence), fear (high arousal and low valence), sadness (low arousal and low valence), and relax (low arousal and high valence) states .
For acquiring EEG signals, the 24-channel EEG traveller is used, which has options of different sampling frequencies and montages for recording. Here, the transverse bipolar montage arrangement is built up according to the 10–20 electrode system for acquiring the EEG signals at 256 Hz sampling frequency. In the 10–20 system, each electrode is represented by a letter and a number, which respectively identifies the lobe and hemisphere where it has to be placed. Corresponding to an impulse-stimulus, the frontal and tem- poral regions of the skull are playing an important role to execute any reaction in the human brain. So, electrode positions FP1, FP2, F3, F4, F7, F8, T3, T4, T5, and T6 EEG-recordings are considered for emotion recognition. The reference and ground electrodes are placed over the forehead one above the other so the disturbance created due to the eye movement was not recorded. The following conditions are the same for the EEG recordings of each subject: the subjects do not have any neurological diseases, not consume alcohol and medicines, and subjects have taken proper sleep last night.
Publication in the Data-set
[1] Anala Hari Krishna, Aravapalli Bhavya Sri, KYVS Priyanka, Sachin Taran, and Varun Bajaj Emotion classification using EEG signals based on tunable-Q wavelet transform IET Science, Measurement and Technology 13(3) 375-380 SCI 10.1049/iet-smt.2018.5237 2018 2.23 Q2 IET
[2] Sachin Taran, Varun Bajaj, Emotion recognition from single-channel EEG signals using a two-stage correlation and instantaneous frequency-based filtering method Computer Methods and Programs in Biomedicine 173 157-165 SCI https://doi.org/10.1016/j.cmpb.2019.03.015 2019 3.632 Q1 Elesvier
[3] Varun Bajaj, Sachin Taran and Abdulkadir Sengur, Emotion classification using Flexible analytic wavelet transform for electroencephalogram signals, Health Information Science and Systems 06:12 1-7 SCImago https://doi.org/10.1007/s13755-018-0048-y, 2018,Q1 Springer
[4]Smith K. Khare, and Varun Bajaj, An Evolutionary Optimized Variational Mode Decomposition for Emotion Recognition, IEEE Sensors Journal, 2020,3.07 Q1 IEEE.
[5]Smith K. Khare, Anurag Nishad, Abhay Upadhyay, and Varun Bajaj, Classification of emotions from EEG signals using time-order representation based on the S-transform and convolutional neural network, Electronics Letters, 2020, 1.232 Q1 IET.
[6] Smith Khare, Varun Bajaj, Time-Frequency Representation and Convolutional Neural Network based Emotion Recognition, IEEE Transactions on Neural Networks and Learning Systems, SCI, 2020, 8.793 Q1 IEEE.
[7] Smith K. Khare, Varun Bajaj, G. R. Sinha, Adaptive Tunable Q Wavelet Transform based Emotion Identification, IEEE Transactions on Instrumentation and Measurement, SCI 10.1109/TIM.2020.3006611, 2020, 3.67 Q1 IEEE
Data-set Available on request:
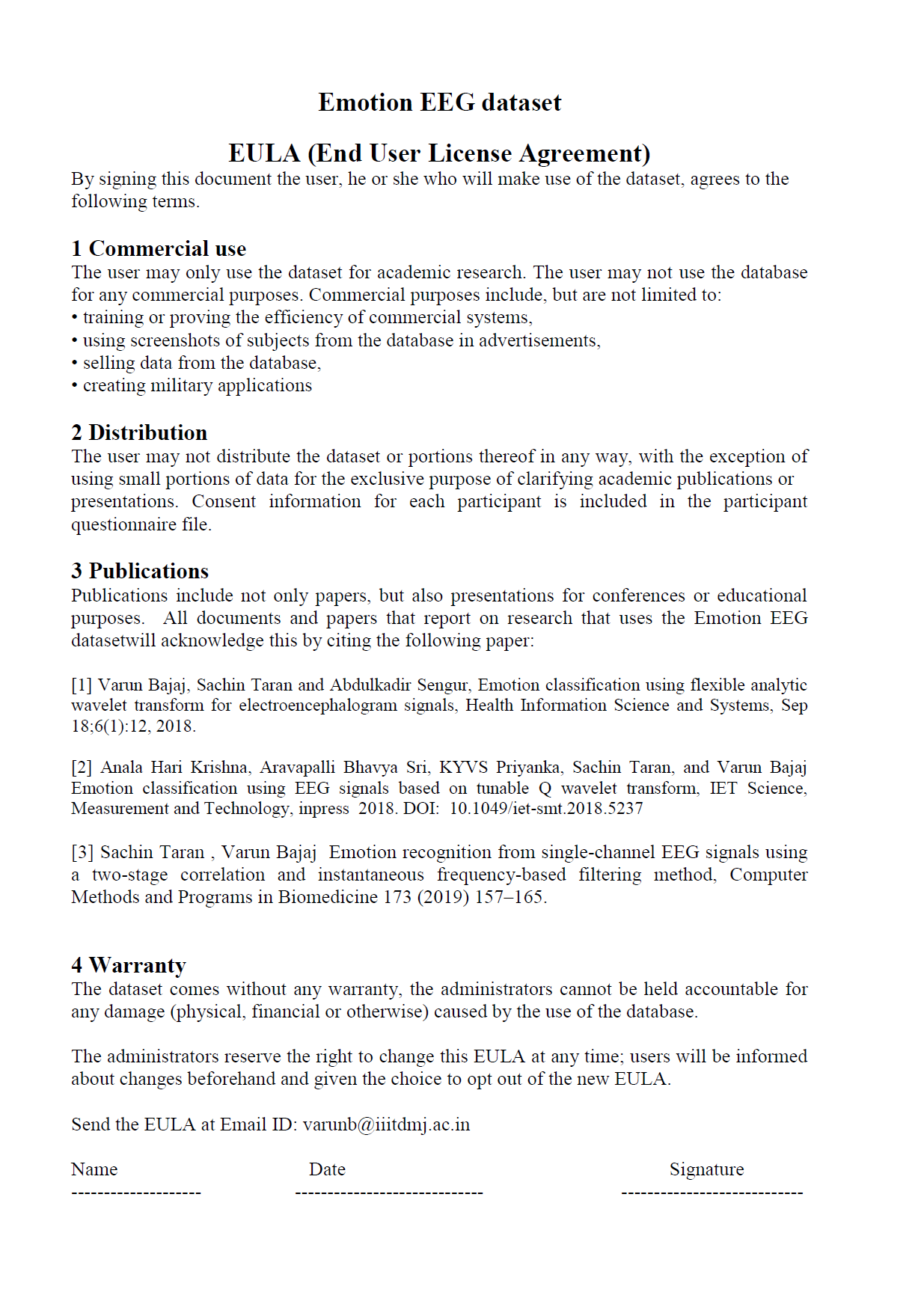